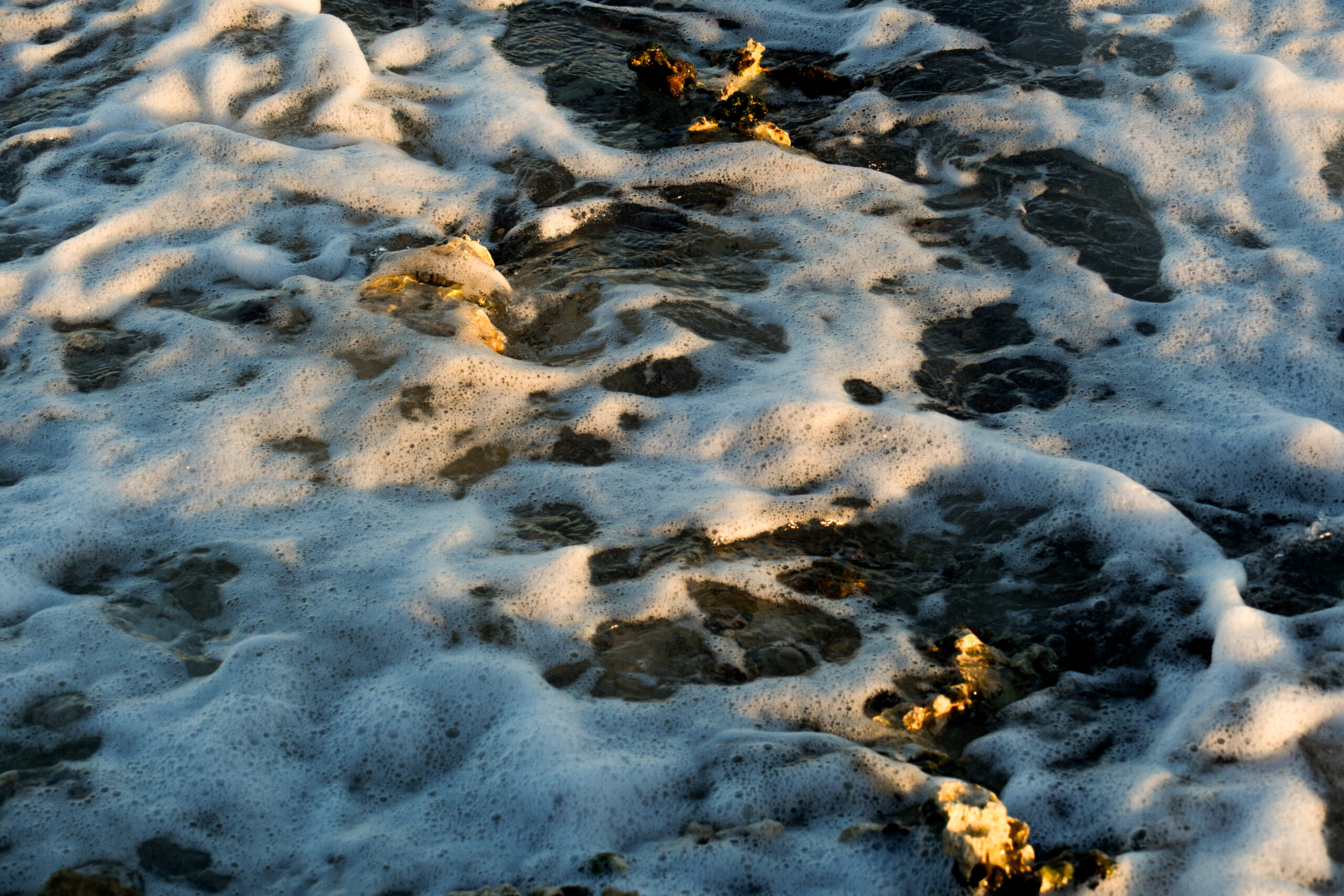
I make products and photographs and software and stockpiles of data.
From my point
of view
This site is a personal portfolio from someone who likes to explore. I mean “explore” quite broadly: I explore cities, landscapes, technologies, markets and ideas. Here, I share some things I see, make, learn or think along the way.
If you’re here to understand me in my professional life, my CV is a good place to start. Otherwise, explore whatever interests you. That’s most of what I do.
Colin Temple
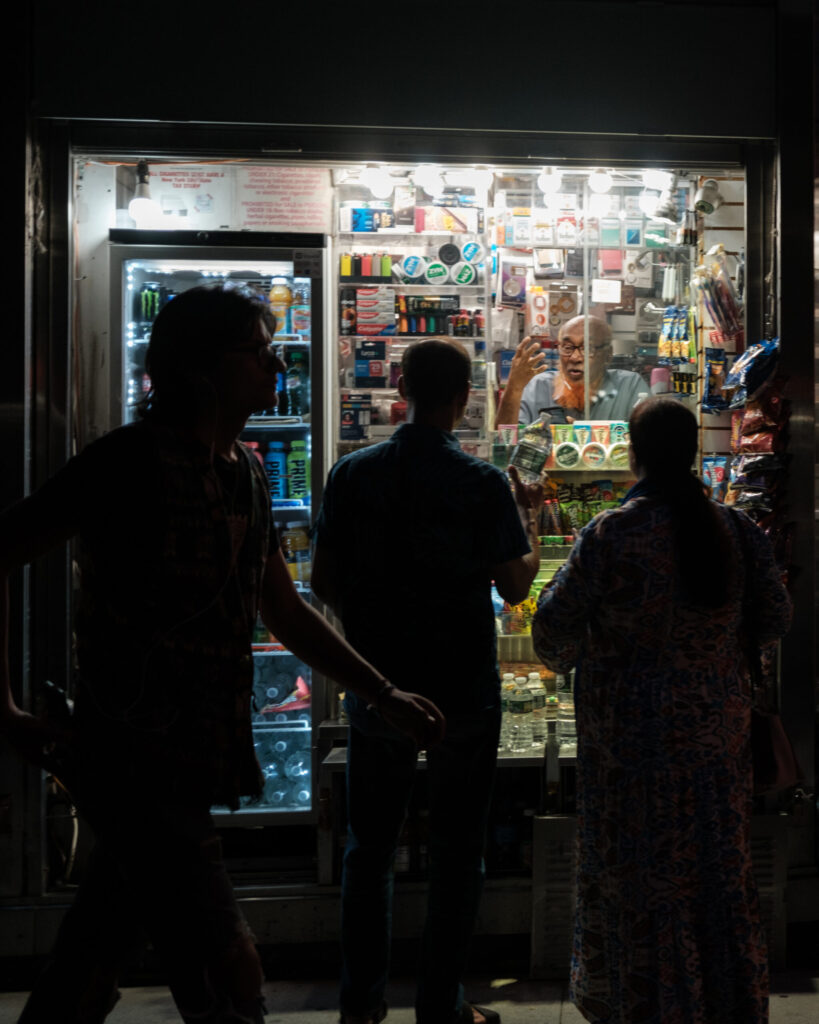
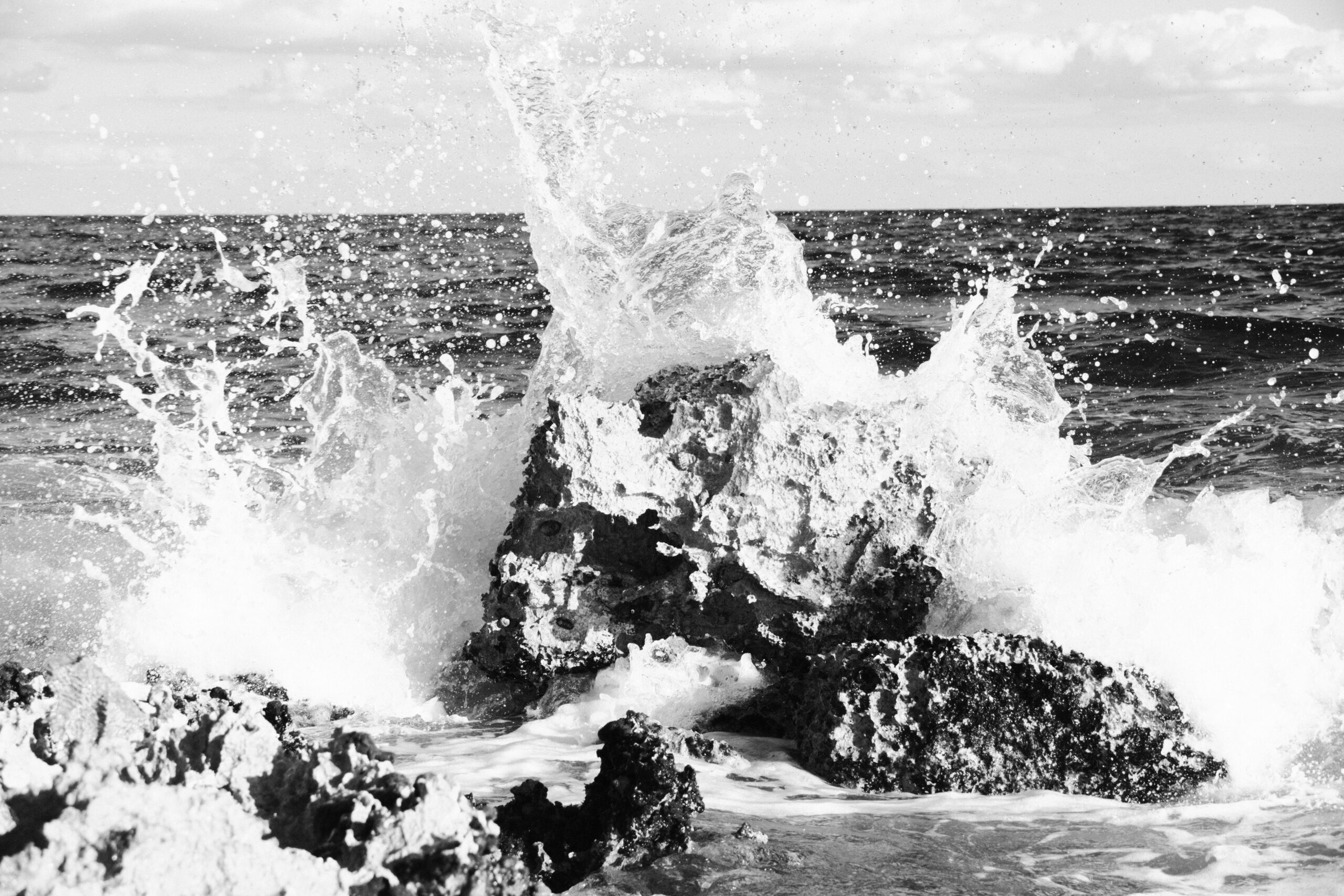
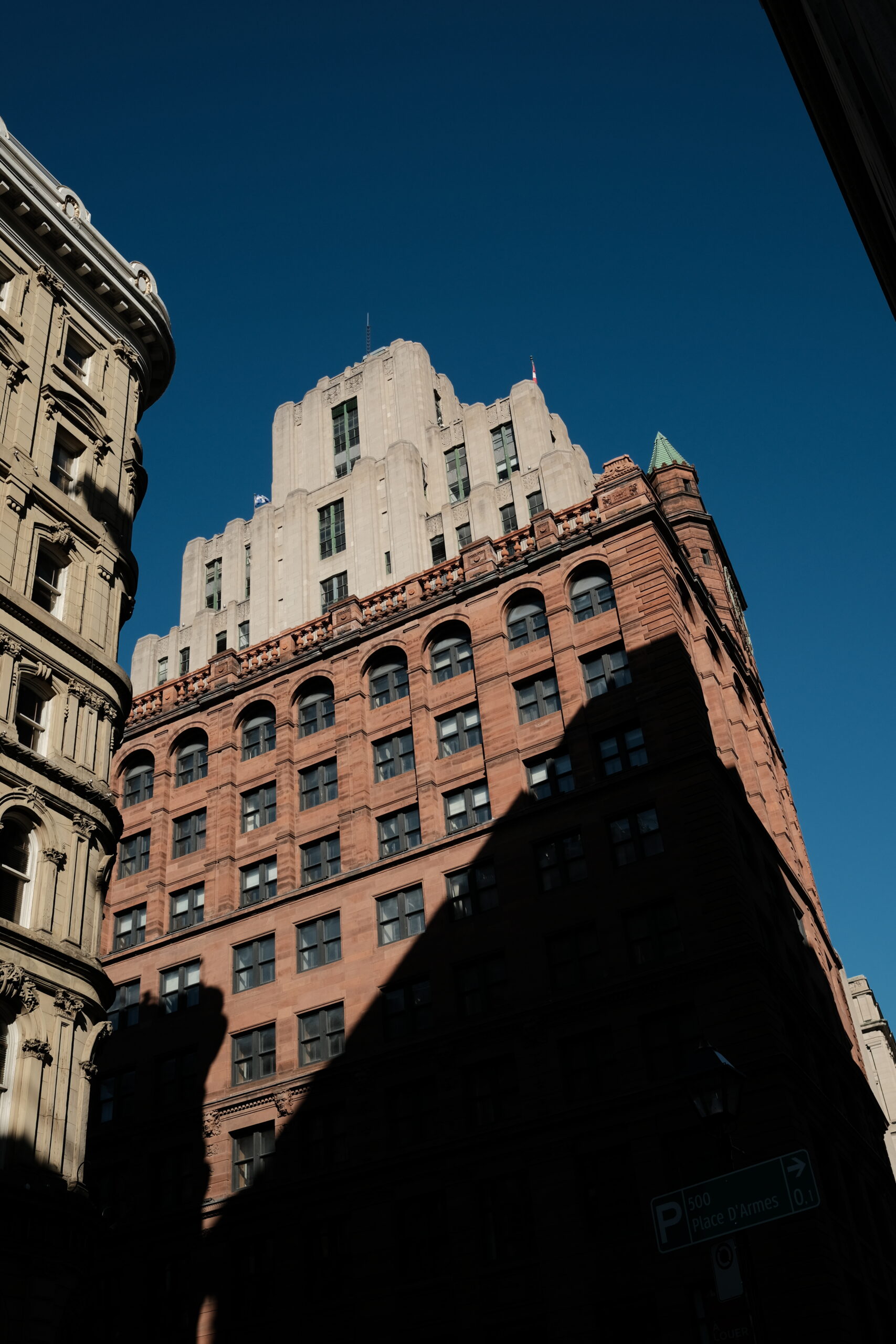
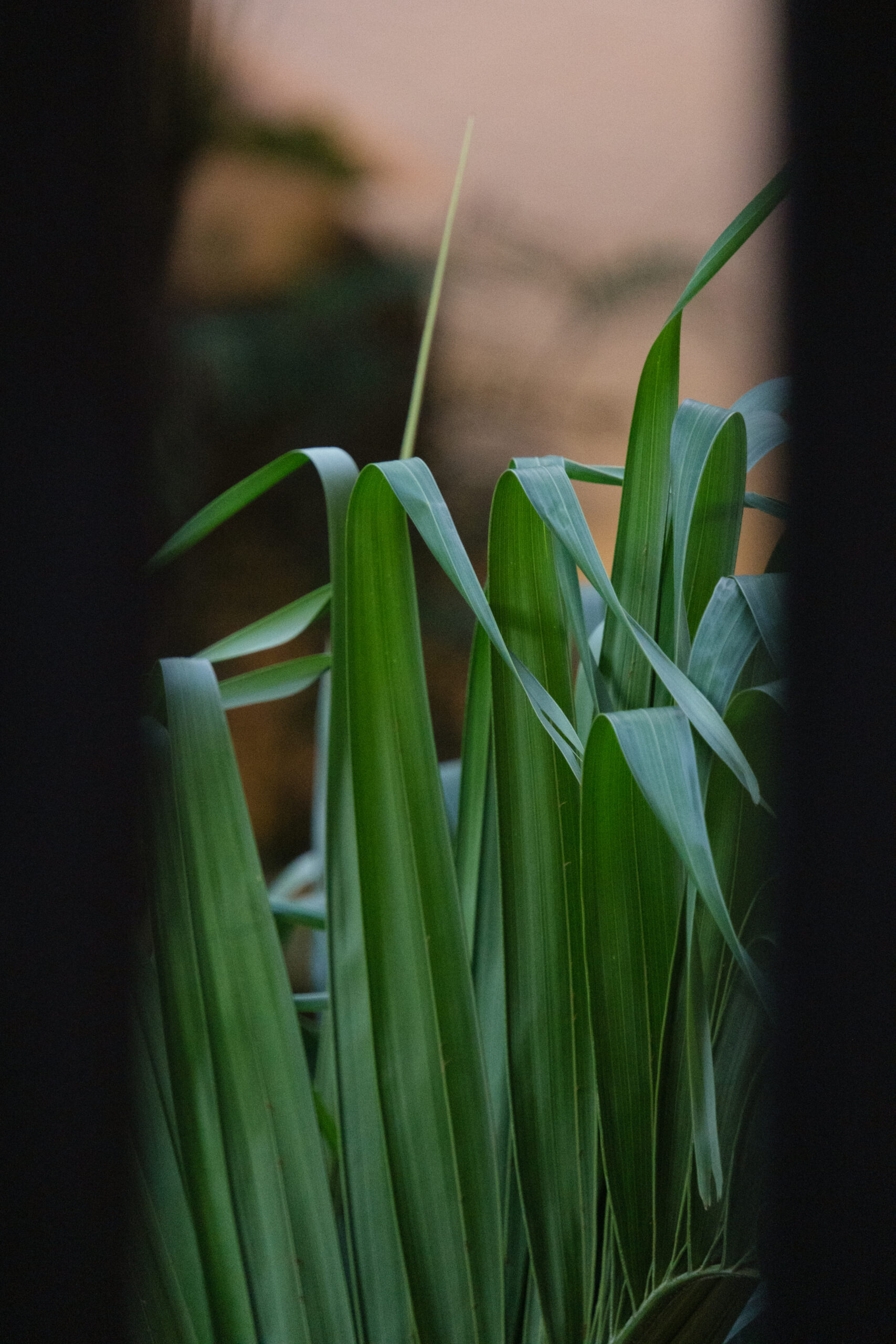
Photographs
Some recent photographs
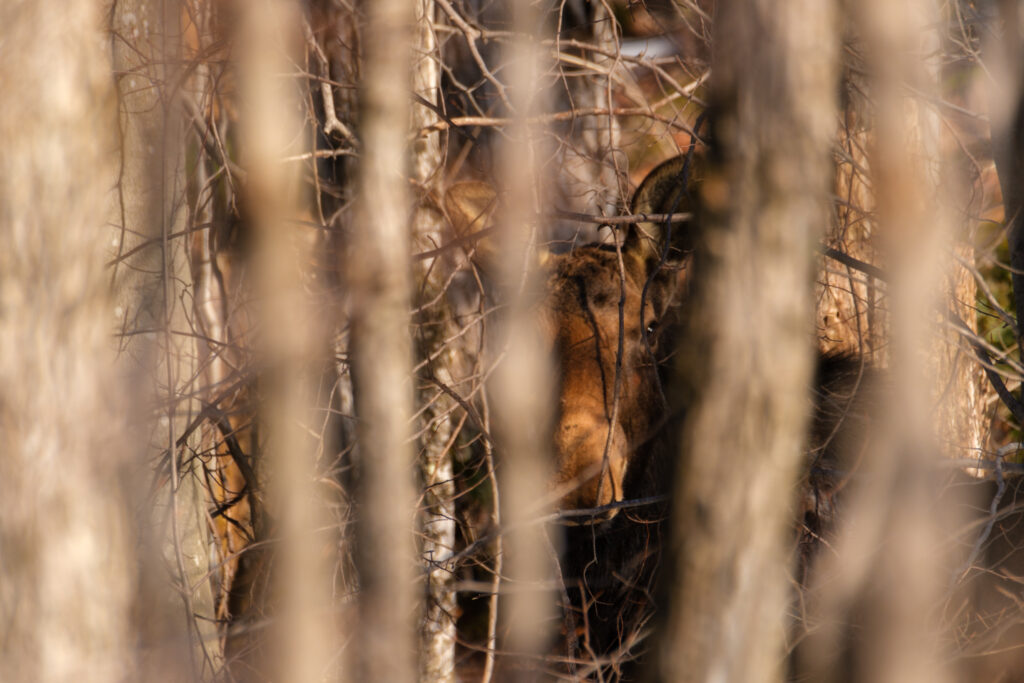
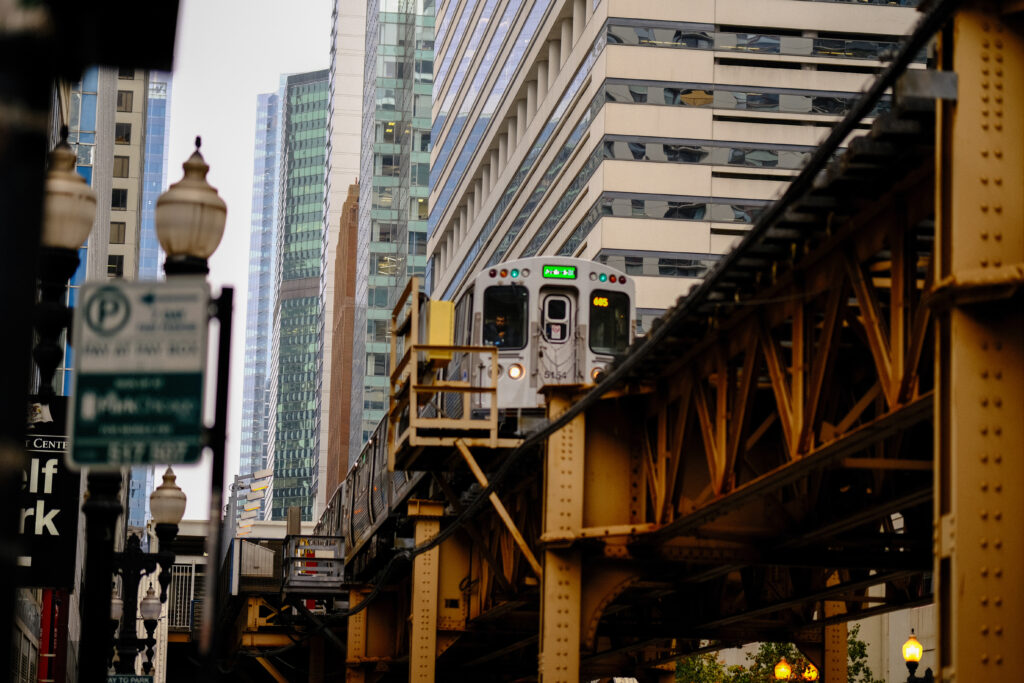
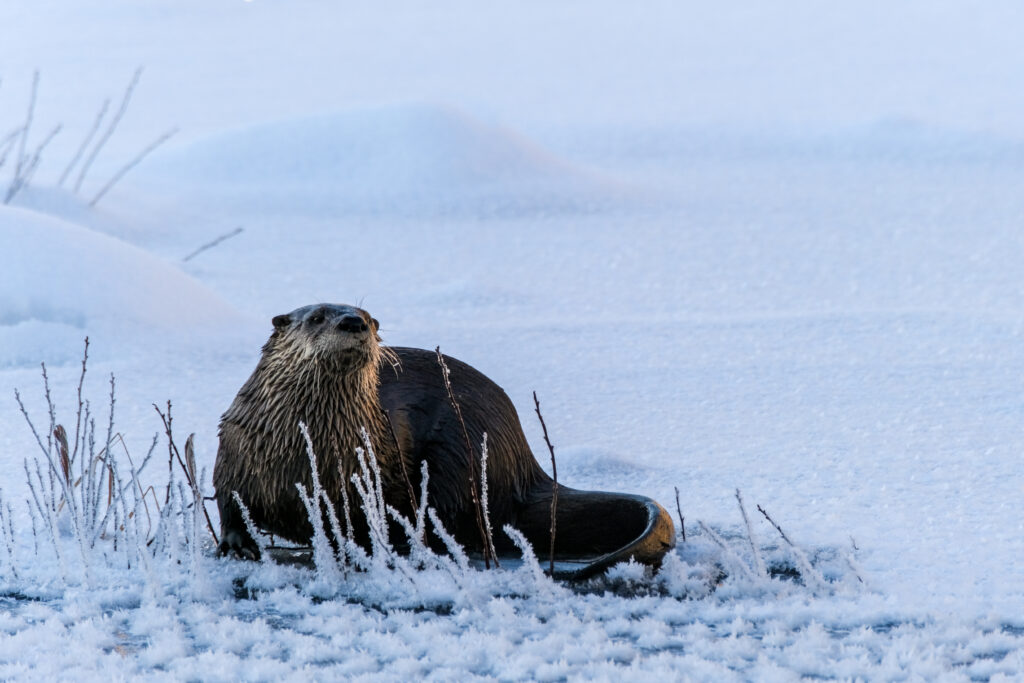
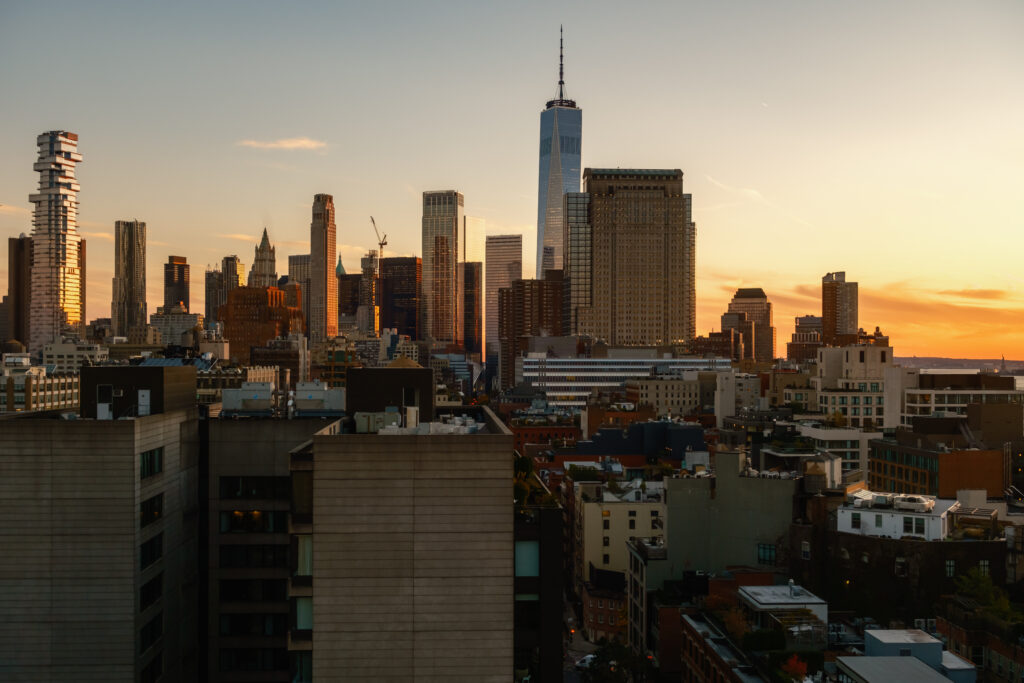
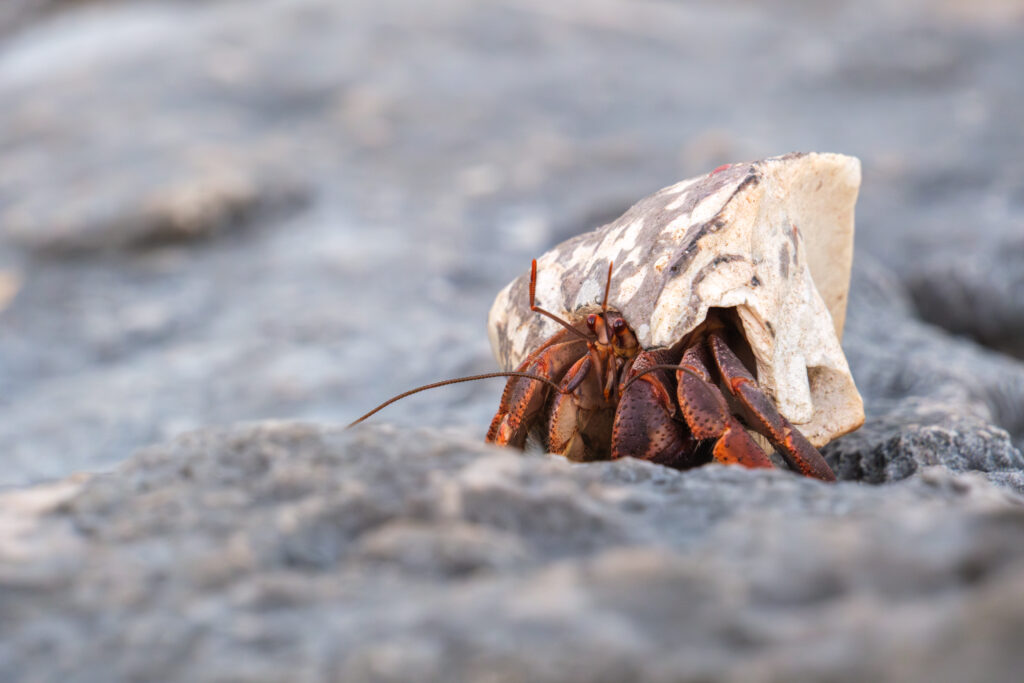